evaluation is based
on high-quality data
technical coordinator
In a nutshell
What this step
is about
During your trial you gathered a lot of different kinds of data with various means (observer, test-bed technical infrastructure, questionnaires etc.). This was done according to your data collection plan. Now plans are always just ideal imaginations of how the reality should work. There are cases in which plans work out as expected, but it is common that deviations occur. These deviations are exactly what we need to identify during the data quality check.
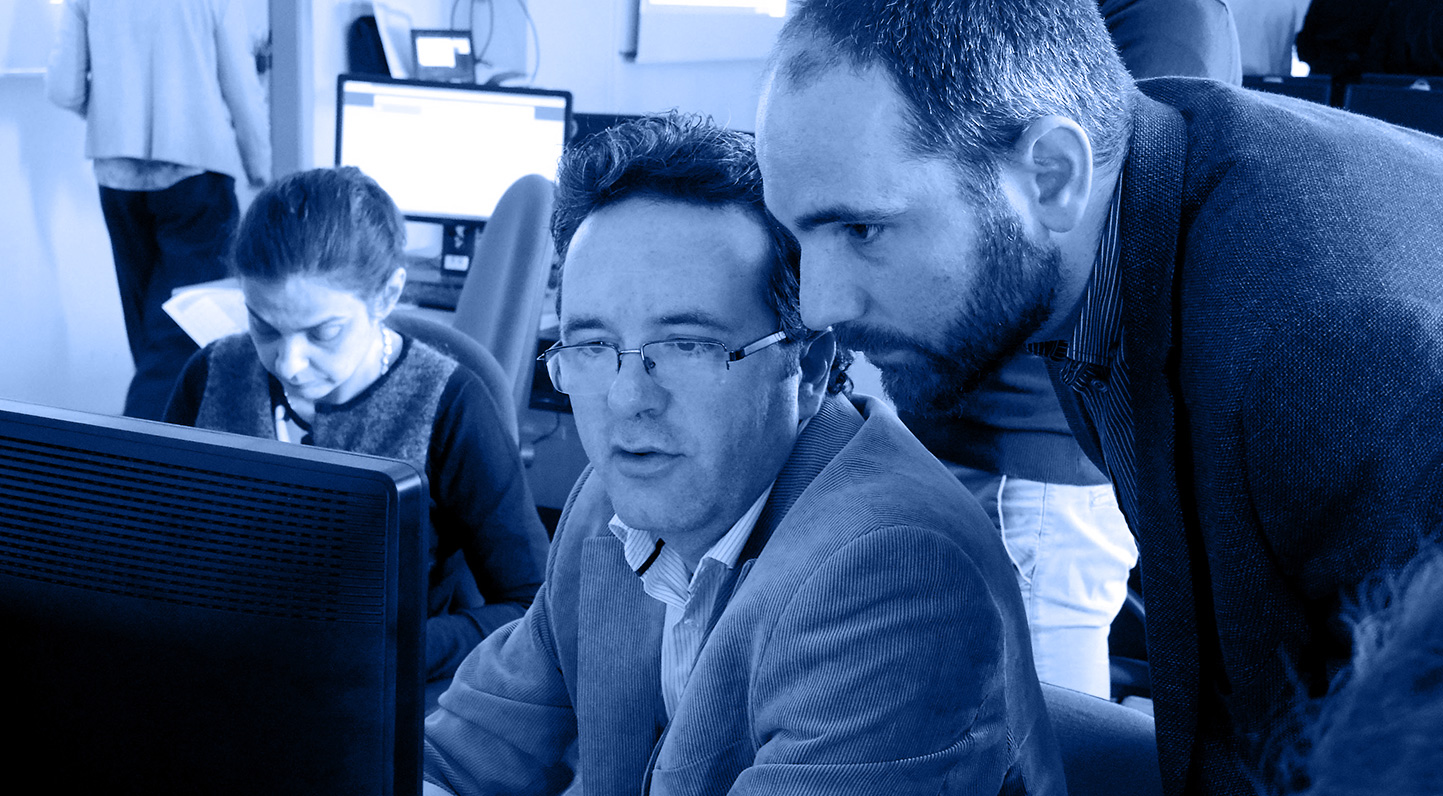
In depth
All you need to know
about this step
First, gather all the data you collected in one place and in the same format. Maybe you want to have it all in one Excel file, maybe you prefer another tool. But make sure you have everything in one place and format it! Do the first check: Is there data missing or broken? If so, is this data critical? If so, think of ways to regain it (repair or maybe ask a participant to have a phone call and fill in a dedicated questionnaire).Even if it is not critical, make sure to indicate where data is missing in your evaluation!
Second, structure your data. Have a look at your data collection plan. Is there a structure to use? Maybe according to role, solution, research question (maybe the 3 dimensions: solution, trial and CM). Now it is easier to see through. Do the second check: Is there data missing or broken? Third, have a closer look at the data quality. Look for patterns. Look for things that don’t fit those patterns. Check why they don’t fit. Are there strong deviations? If so, try to find more data related to the aspect (maybe in the test-bed technical infrastructure?). If there is no way to improve the data, indicate in the evaluation that the conclusions on this can only be limited. Fourth, create a data set for your analysis. Exclude irrelevant or poor quality data, but indicate that you have done that!
Methods
Structuring & organising,
societal impact assessment,
research ethics
Tools
After action review tool,
observer support tool, solutions,
Excel, admin tool and security,
extra developer tools
Input
Raw data
Output
“Clean” data set
Checklist
- Data completeness checked
- Data quality checked
- Data verified
- Data structured in a preliminary way